
Editorials
Use of AI in Family Medicine Publications: A Joint Editorial From Journal Editors
Am Fam Physician. 2025;111(1):6-9
Author disclosure: No relevant financial relationships.
There are multiple guidelines from publishers and organizations on the use of Artificial Intelligence (AI) in publishing.1–5 However, none are specific to family medicine. Most journals have some basic AI use recommendations for authors, but more explicit direction is needed, as not all AI tools are the same.
As family medicine journal editors, we want to provide a unified statement about AI in academic publishing for authors, editors, publishers, and peer reviewers based on our current understanding of the field. The technology is advancing rapidly. While text generated from early large language models (LLMs) was relatively easy to identify, text generated from newer versions is getting progressively better at imitating human language and more challenging to detect. Our goal is to develop a unified framework for managing AI in family medicine journals. As this is a rapidly evolving environment, we acknowledge that any such framework will need to continue to evolve. However, we also feel it is important to provide some guidance for where we are today.
DEFINITIONS
AI is a broad field where computers perform tasks that have historically been thought to require human intelligence. LLMs are a recent breakthrough in AI, allowing computers to generate text that seems like it comes from a human. LLMs deal with language generation, while the broader term generative AI can also include AI-generated images or figures. ChatGPT is one of the earliest and widely used LLMs, but other companies have developed similar products. LLMs “learn” to do a multifaceted analysis of word sequences in a massive text training database and generate new sequences of words using a complex probability model. The model has a random component, so responses to the exact same prompt submitted multiple times will not be identical. LLMs can generate text that looks like a medical journal article in response to a prompt, but the article's content may or may not be accurate. LLMs may “confabulate,” generating convincing text that includes false information.6–8 LLMs do not search the internet for answers to questions. However, they have been paired with search engines in increasingly sophisticated ways. For the rest of this editorial, we will use the broad term AI synonymously with LLMs.
ROLE OF LARGE LANGUAGE MODELS IN ACADEMIC WRITING AND RESEARCH
As LLM tools are updated and authors and researchers become familiar with them, they will undoubtedly become more functional in assisting the research and writing process by improving efficiency and consistency. However, current research on the best use of these tools in publication is still lacking. A systematic review exploring the role of ChatGPT in literature searches found that most articles on the topic are commentaries, blog posts, and editorials, with little peer-reviewed research.9 Some studies have demonstrated benefit in narrowing the scope of literature review when AI tools were applied to large data sets of studies and prompted to evaluate them for inclusion based on the title and abstract. Another paper reported that AI had 70% accuracy in appropriately identifying relevant studies compared with human researchers and may reduce time and provide a less subjective approach to literature review.10–12 When used to assist with writing background sections, LLMs' writing was rated the same, if not better, than that of human researchers, but citations were consistently false in another study.13 LLM models are frequently deficient in providing “real” papers and correctly matching authors to their own papers when generating citations and therefore are at risk of creating fictitious citations that appear convincing despite incorrect information, including DOI numbers.6,14
Studies evaluating the perceptions of AI use in academic journals and evaluating the strengths and weaknesses of the tools revealed no agreement on how to report the use of AI tools.15 There are many tools; for example, some are used to improve grammar, and others generate content, yet parameters on substantive use vs non-substantive use are lacking. Furthermore, current AI detection tools cannot adequately distinguish use types.15 Reported benefits include reduced workload and the ability to summarize data efficiently, whereas weaknesses include variable accuracy, plagiarism, and deficient application of evidence-based medicine standards.7,16
Guidelines on appropriate AI use exist, such as the “Living Guidelines on the Responsible Use of Generative AI in Research” produced by the European Commission.17 These guidelines include steps for researchers, organizations, and funders. The fundamental principles for researchers are to maintain ultimate responsibility for content; apply AI tools transparently; ensure careful evaluation of privacy, intellectual property, and applicable legislation; continuously learn how best to use AI tools; and refrain from using tools on activities that directly affect other researchers and groups.17 While these are helpful starting points, family medicine publishers can collaborate on best practices for using AI tools and help define substantive reportable use while acknowledging the current limitations of various tools and understanding that they will continue to evolve. Family medicine journals do not have unique AI needs compared with other journals, but the effort of all the editors to jointly present principles related to AI is a unique model.
GUIDANCE FOR USE OF LLMS/AI IN FAMILY MEDICINE PUBLICATIONS
The core principles of scientific publishing will remain essentially unchanged by AI. For example, the criteria for authorship will remain the same. Authors will still be required to be active participants in conceptualizing and producing scientific work; writers and editors of manuscripts will be held accountable for the product (Table 1).
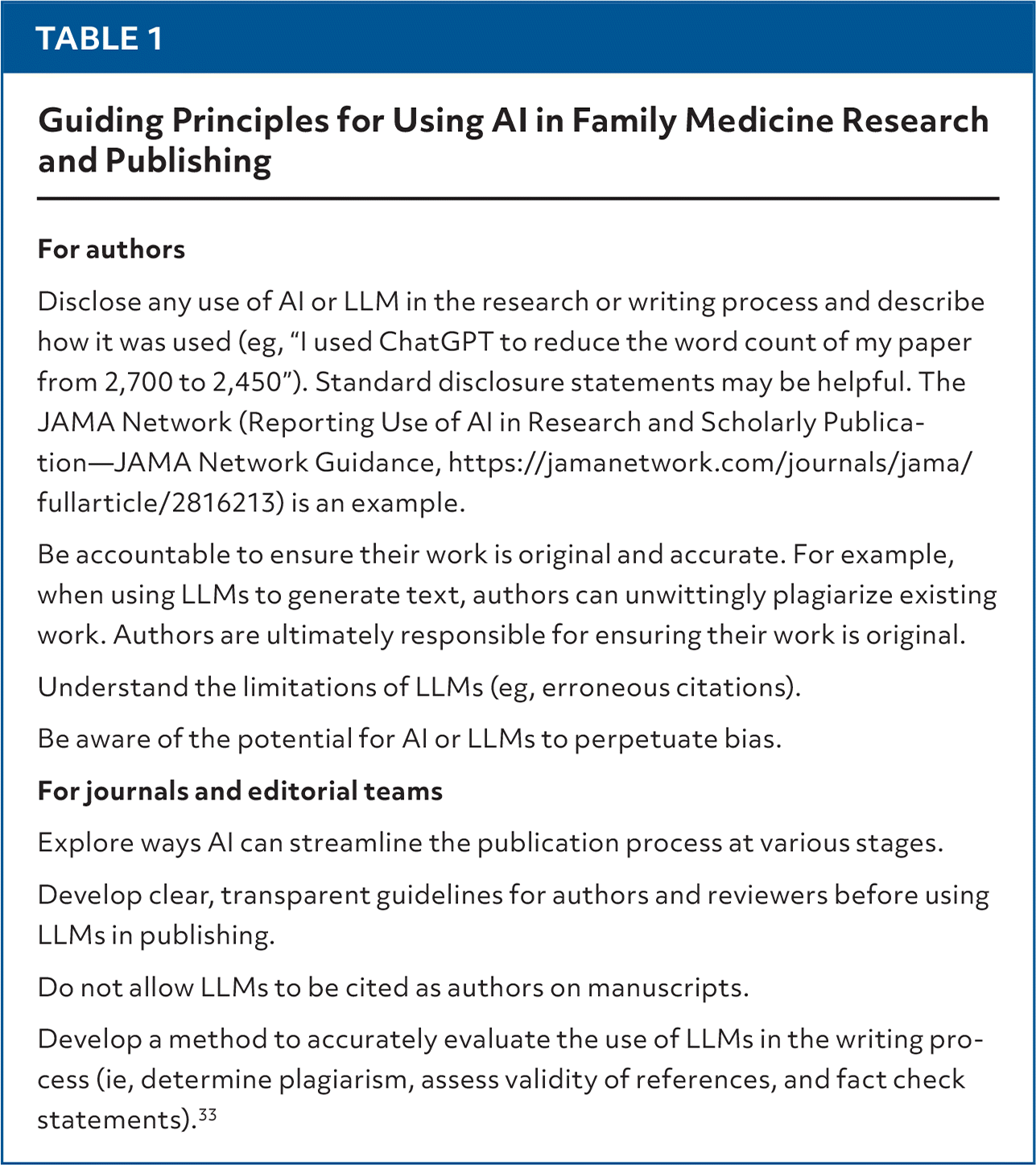
For authors |
Disclose any use of AI or LLM in the research or writing process and describe how it was used (eg, “I used ChatGPT to reduce the word count of my paper from 2,700 to 2,450”). Standard disclosure statements may be helpful. The JAMA Network (Reporting Use of AI in Research and Scholarly Publication—JAMA Network Guidance, https://jamanetwork.com/journals/jama/fullarticle/2816213) is an example. |
Be accountable to ensure their work is original and accurate. For example, when using LLMs to generate text, authors can unwittingly plagiarize existing work. Authors are ultimately responsible for ensuring their work is original. |
Understand the limitations of LLMs (eg, erroneous citations). |
Be aware of the potential for AI or LLMs to perpetuate bias. |
For journals and editorial teams |
Explore ways AI can streamline the publication process at various stages. |
Develop clear, transparent guidelines for authors and reviewers before using LLMs in publishing. |
Do not allow LLMs to be cited as authors on manuscripts. |
Develop a method to accurately evaluate the use of LLMs in the writing process (ie, determine plagiarism, assess validity of references, and fact check statements).33 |
Authors must still cite others' work appropriately when creating their current scientific research. Citing works will likely change over time as AI use in publishing matures. It is impossible to accurately list all sources used to train a given AI product. However, it would be possible to cite where a fact came from or who originated a particular idea. Similarly, authors will still need to ensure that their final draft is sufficiently original and that they have not inadvertently plagiarized others' works.1,18 Authors must be well-versed in the existing literature of a given field.
IMPACT ON DEI EFFORTS
Because LLMs model text generation on a training data set, there is an inherent concern that they will discover biased arguments and then repeat them, thereby compounding bias.19 Because LLMs mimic human-created content, and there is a preponderance of biased, sexist, racist, and other discriminatory content on the internet, this is a significant risk.20 Some companies now work in the LLM/AI space to eliminate biases from these models, but they are in their infancy. Equality AI, for example, is developing “responsible AI to solve healthcare's most challenging problems: inequity, bias and unfairness.”21 More investment is necessary to further remove bias from LLM/AI models. While authors have touted AI and LLMs as bias elimination tools, the fact that the results of bias elimination tools are not reproducible with any consistency has scholars questioning their utility. Successful deployment of an unbiased LLM/AI tool will depend on carefully examining and revising existing algorithms and the data used to train them.22 Excellent, unbiased algorithms have not been developed but might be in the future.23 AI tools can be used as a de facto editorial assistant that may help globalize the publication process by helping non-native English speakers publish in English-language journals.
FUTURE DIRECTIONS
The use of LLMs and broader AI tools is expanding rapidly. There are opportunities at all levels of research, writing, and publishing to use AI to enhance our work. A key goal for all family medicine journals is to require authors to identify the use of LLMs and assure that the LLMs used provide highly accurate information and mitigate the frequency of confabulation. Research is ongoing to develop methods to determine the accuracy of LLMs output.24 Editors and publishers must continue to advocate for accurate tools to validate the work of LLMs. Researchers should assess the performance of tools that are used in the writing process. For example, they should study the extent to which LLMs plagiarize, provide false citations, or generate false statements. They should also study tools that detect these events.
AI tools are already being used by some publishers and editors to do initial screens of manuscripts and to match potential reviewers with submitted papers. The complex interplay between AI tools and humans is evolving.25 While AI will likely not replace human researchers, authors, reviewers, or editors, it continues to contribute to the publication process in myriad ways. We want to know more: “How can LLMs contribute to the publication process?” “Can authors ask LLMs to do literature searches or draft a paper?” “Can we train AI to contribute to a revision of a paper or to review a paper?” Probably yes, but we must scrutinize any AI-generated references and we likely cannot train AI to evaluate conclusions or determine impact of a specific paper in the field. Family medicine journals are publishing important papers on AI — not only about its use in research and publishing, but also about its use in clinical practice,26–32 and this editorial is a call for more scholarship in this area.
The authors would like to acknowledge Dan Parente, Steven Lin, Winston Liaw, Renee Crichlow, Octavia Amaechi, Brandi White, and Sam Grammer for their helpful suggestions.
Note: This article is being published simultaneously in American Family Physician, Annals of Family Medicine, BMJ Family Medicine and Community Health, Canadian Family Physician, Evidence-Based Practice, Family Medicine, FP Essentials, FPM, Journal of the American Board of Family Medicine, and PRiMER.