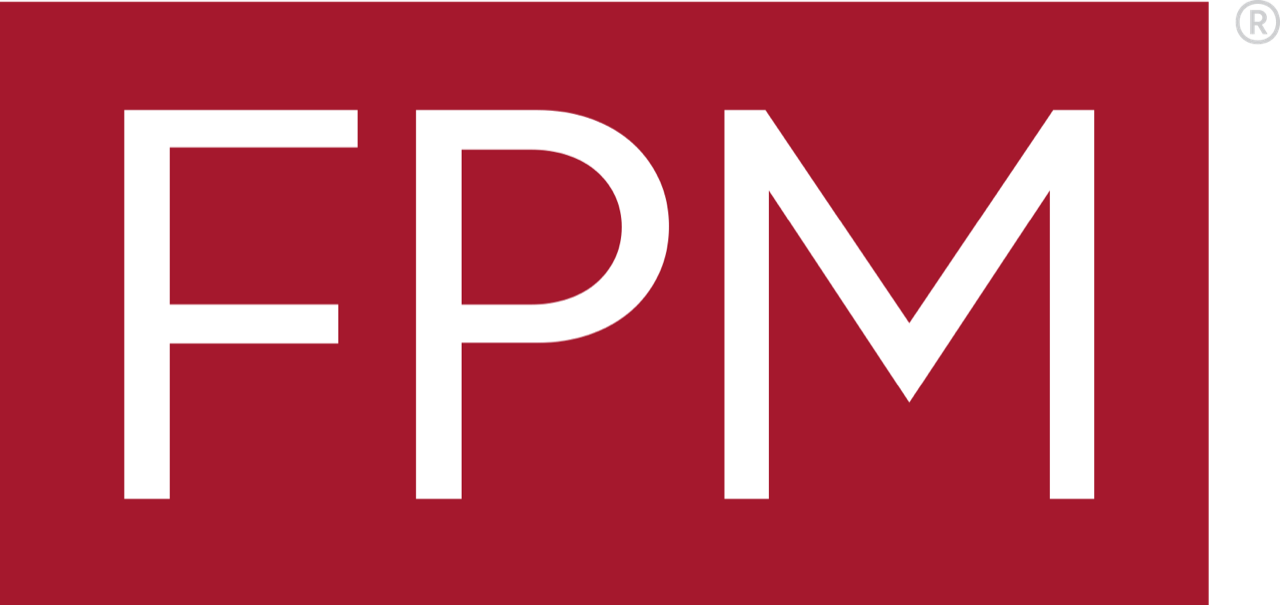
Programs like ChatGPT have the potential to greatly diminish your administrative burden. But how do you get started — and can you trust them?
Fam Pract Manag. 2024;31(2):27-31
Author disclosure: no relevant financial relationships.

Near the end of 2022, a group called OpenAI launched ChatGPT, a large language model (LLM) artificial intelligence (AI) chatbot. It may have seemed like a novelty at first (e.g., “Write a poem about Medicare in the style of Hamilton”), but it soon became apparent that ChatGPT and AI models like it could have huge implications for education, business, and even medicine.
By January 2023, ChatGPT had become the fastest-growing consumer software application in history, reaching 100 million users in just two months (TikTok held the previous record at nine months).1 Before the end of the year, other companies launched similar products, such as Google's Bard (now Gemini) and Microsoft's Bing Chat (now Copilot).
AI is here to stay and will likely become more embedded in our daily lives in the coming years. If used properly, it could be a tremendous boon to primary care physicians, potentially ridding us of administrative tasks that are a leading source of burnout.2 But, as with any new technology, there are downsides. This article seeks to illuminate some of the ways AI can help primary care practices now and in the near future — and some of the ways AI could be downright dangerous.
KEY POINTS
New artificial intelligence (AI) systems such as ChatGPT can reduce administrative burden, but their current shortcomings make it inadvisable to use them to aid clinical decision making.
Tasks AI can help with now include drafting prior authorization requests, rewriting medicolegal forms in more patient-friendly language, and explaining normal test results.
Proprietary or HIPAA-protected information should only be submitted to closed, private AI systems, not open systems such as ChatGPT.
Subscribe
From $95- Immediate, unlimited access to all FPM content
- More than 36 CME credits/year
- AAFP app access
- Print delivery available
Issue Access
$39.95- Immediate, unlimited access to this issue's content
- CME credits
- AAFP app access
- Print delivery available
Article Only
$25.95- Immediate, unlimited access to just this article
- CME credits
- AAFP app access
- Print delivery available